WEEK 4: MEASURING IMPACT
- Rigzom Wangchuk
- Nov 7, 2021
- 3 min read
Updated: Nov 10, 2021
Measuring impact is a core component of evidence-based policy making. We measure impact for two primary reasons: (1) external accountability to beneficiaries, donors, investors, partners, and peers. (2) internal accountability to improve our own programming and to monitor if resources are being spent optimally.
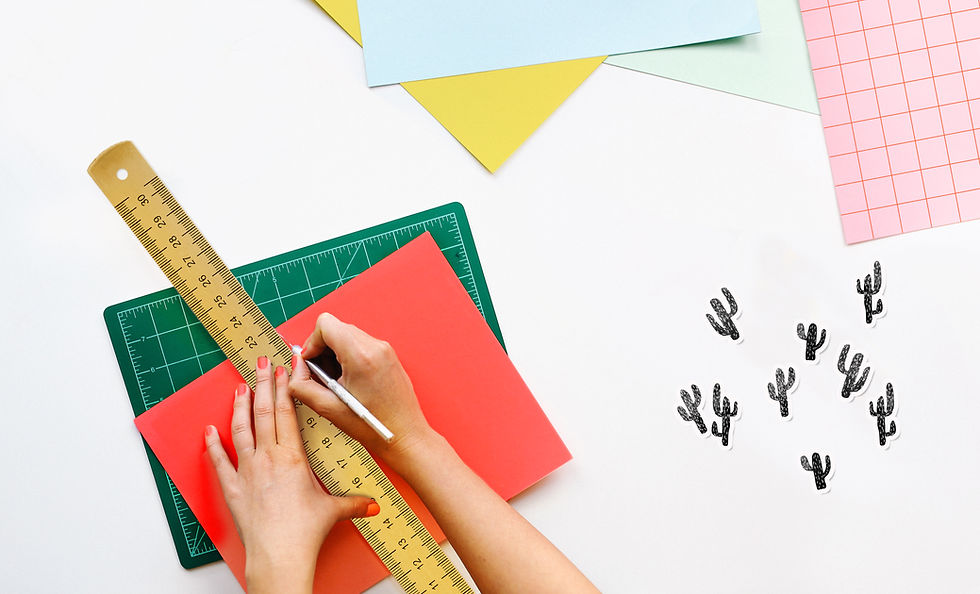
From my experience of working with programs and organisations across the world, I have come to realise that, more often than not, organisations are pressurised to act fast when faced with crisis or from external pressure. This was especially the case during the COVID-19 pandemic and the challenge the City Hall of Recife faced. While there was good data available on vulnerable people, the City Hall had to act fast. The City Hall is also equipped with manpower who have the skills to analyse data. With this respect, I felt my internship with the City Hall was well supported. However, we also needed to catch up with a program that had already started to set up and start implementing the evaluation framework.
Here are a few lessons I learned during this process:
1. A clear theory of change is needed!
A question I kept asking myself and the team I was working with was, "what is the ultimate impact you are trying to see?" For me, the following chart was helpful to visualise what type of data we need to collect at each stage to measure the flow of impact we hope to see. Since CredPOP was focusing on women entrepreneurs and economic empowerment, we wanted to focus on understanding how women were using their profit. How much of it went to reinvesting into their business for growth vs. how much contributed to their economic independence. Are women able to break even and pay back loans? A long-term impact we considered measuring was also whether economic independence had an impact on domestic violence.
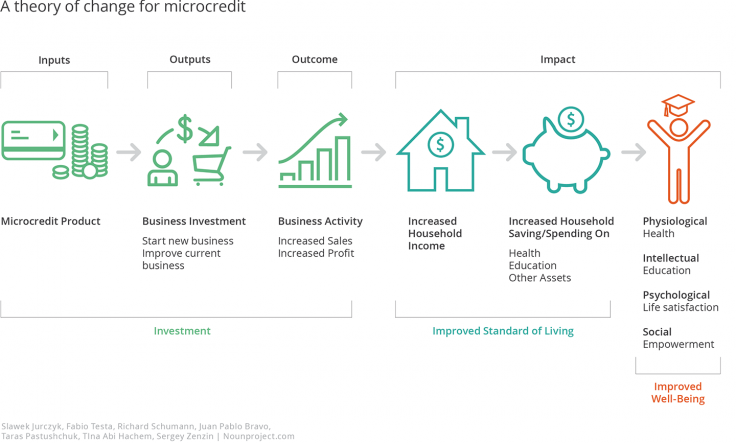
Source: JPAL
2. Establish metrics to measure your dependent and independent variables.
The Theory of Change helped us understand what our dependent variables should be. For example, at the output stage, our dependent variable was (1) if a new entrepreneur, whether a business was started or not upon receiving credit; and (2) if an old entrepreneur, whether credit was reinvested in the business and for what purpose. At the outcome stage, our dependent variable was measuring the size per unit time business sales and profit. This also made us realise that we needed to tag and track business sales and income data for existing business separately, and possibly go back to get more data on business figures pre-COVID.
Since the intervention was not just credit but also capacity building ones, we also had to decide how the additional services would be allocated and measured.
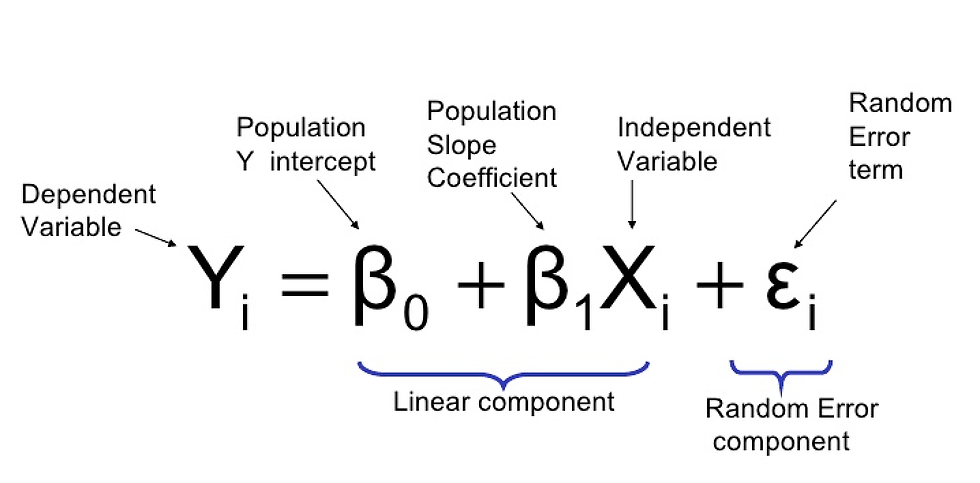
Source: Towards Data Science
3. Who are your comparison groups?
This was a point of confusion because this was inherently linked to the type of data available, data that needed to be collected and how often, and what the appropriate methodology to use was. We couldn't do a male vs. women comparison as the credits were only given to women and some vulnerable people. The next question was if we should compare women who got the credit vs. who didn't? This was ruled out due to self-selection bias of the type of women who seek credit. An RCT was ethically ruled out due to the gravity of the situation on the ground. And so on and so froth.
Finally, we decided to create the comparison groups by cohorts who received credit at different times. By comparing those waiting to get credit and those who have received credit at different points in time, we thought it would be feasible to capture impact.
4. Statistical method of choice to measure impact.
I found Impact Evaluation in Practice by the World Bank and IBD a very useful guide to understand which framework to use given what you have at hand and what type of data you are able to collect/generate:
Randomised Controlled Trials (RCTs) made popular by Easter Duflo and Abhijeet Banerjee of J-PAL/IPA
Regression Discontinuity Design
Difference-in-Difference
Matching
Instrumental Variable (IV) - very difficult to do as IVs are a rare specifies
Some additional resources:
USAID's Technical Note on Impact Evaluations and Toolkit.
Comments