WEEK 5: IMPROVING IMPLEMENTATION USING DATA
- Rigzom Wangchuk
- Nov 7, 2021
- 3 min read
Updated: Nov 10, 2021
I have never experienced a data collection phase that is smooth. My internship at the City Hall of Recife was no exception.
One of my early contributions to my internship was realising we were not collecting the right data or using the right instrument to track targeting of the program. Assessing targeting accuracy is based on two key measures:
1. Leakage (or inclusion error) – when non-intended beneficiaries receive program benefits. This for the CredPOP program were male who were not vulnerable.
2. Under-coverage (or exclusion error) – when intended beneficiaries do not receive program benefits. In CredPOP, this lack of outreach to identified women or vulnerable persons.
It took us a while to realise that there were duplicates in unique IDs as well as the equivalent of SSN in Brazil. I realised this when I was trying to merge data from the first batch of loans dispatched against the application data to run some analysis. This was a system issue that needed retraining of data collector (the first point of contact with the beneficiary) and checking, validating, and fixing at the backend.
For the City Hall, targeting by location was also very important as certain neighbourhoods of Recife have higher concentration of economically vulnerable families and individuals. The following is an initial heat map of applicants I created to track under-coverage and inform out reach for better targeting. There were many such instruments that were being used to check targeting and better understand if implementation gaps or opportunities for improvement.
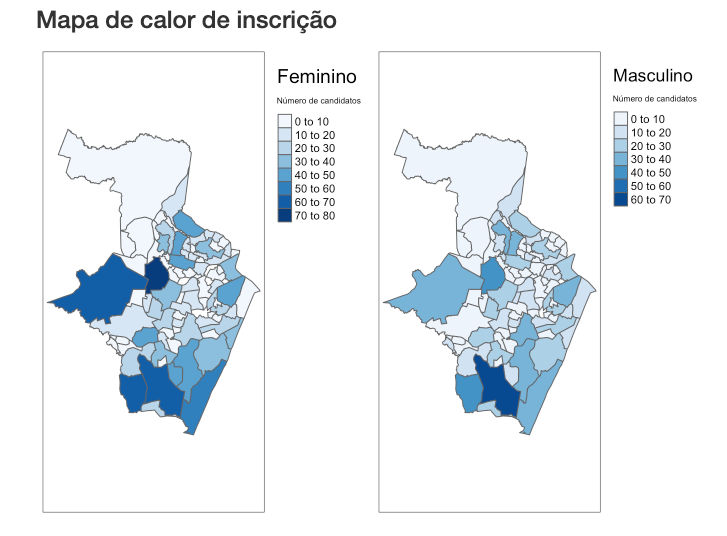
Some lessons I have learned from my internship and prior experience collecting primary data:
Create a pre-analysis plan based on your theory of change. You should have a vision of the analysis you want to conduct and map it to the data frame you need to collect in order to conduct the analysis.
Design your survey questionnaire based on the pre-analysis plan as well as realities on the ground. A pre-analysis plan is needed in order to decide what type of data needs to be collected in numeric versus categorical form. In questions with categorical options, you need to decide which ones you want as single response vs. allowing for multi-response. Sequencing of sections and questions need to follow a logical manner that facilitates the interview to reduce gaming of questions/sections (especially if they’re questions that are testing behavioural patterns).
Length of survey: There are many ways of making questionnaires efficient (such as incorporating skip patterns and ensuring mandatory response to questions to reduce missing data) and technology enables us to this better now. Survey length is a binding constraint to quality data as respondents have opportunity cost to the time they give you.
Simple colloquial translation is best instead of formal translations that leave a lot of room for interpretation. Surveyors should be trained to ask questions in more ways than one.
During data collection, a subset of questions should be selected for timely monitoring where you would expect large variation or clustering.
Have codes for daily monitoring ready ahead of time. This includes checking for duplicates, outliers, frequency of answers like "don't know" and "N/A."
Plan ahead and anticipate anything and everything that could go wrong to mitigate as many issues ahead of time as possible.
Have an open attitude towards learning and improving.
PILOT! PILOT! PILOT! And include qualitative interviews during pilot.
Setting aside budget for a pilot or two when writing project proposals is very crucial.
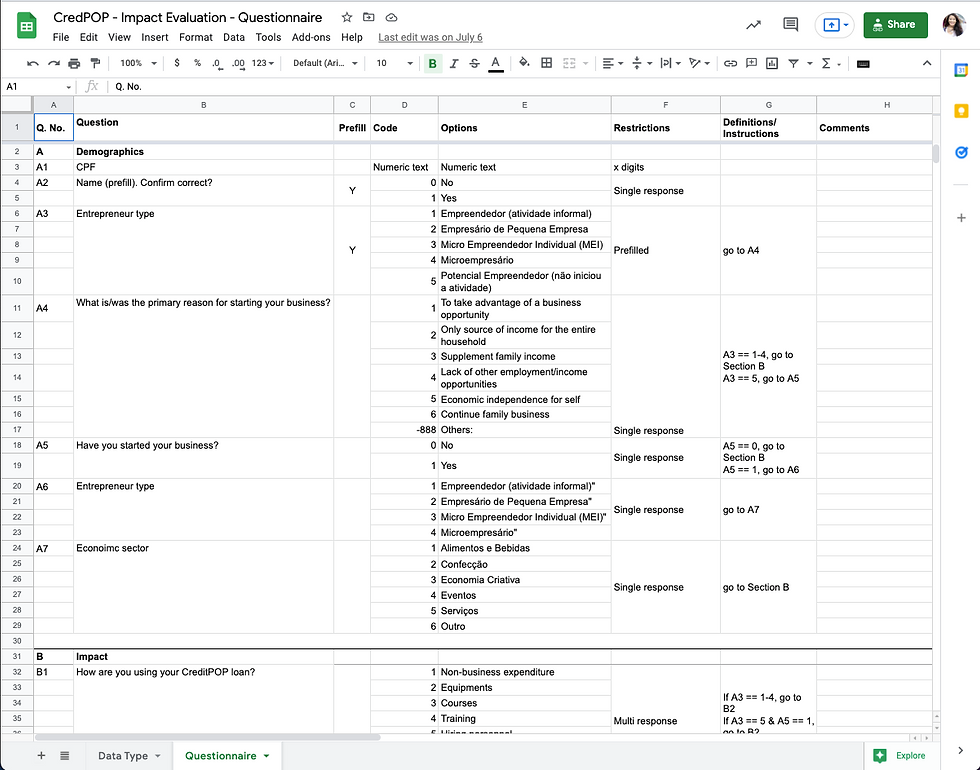
Additional resources for data collection management that I have found helpful in my experience:
1. J-PAL
Comments